Comparative analysis of the machine learning models determining COVID-19 patient risk levels
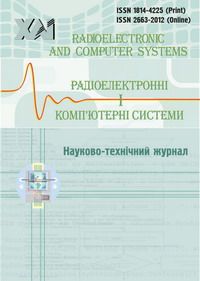
View/Open
Date
2023Author
Bazilevych, K.
Kyrylenko, O.
Parfenyuk, Y.
Krivtsov, S.
Meniailov, I.
Kuznietcova, V.
Chumachenko, D.
Metadata
Show full item recordAbstract
The COVID-19 pandemic has posed unprecedented challenges to global healthcare systems, emphasizing the need for predictive tools for resource allocation and patient care. This study delves into the potential of machine learning models to predict the risk levels of COVID-19 patients using a comprehensive dataset. This study aimed to evaluate and compare the efficacy of three distinct machine learning methodologies – Bayesian Criterion, Logistic Regression, and Gradient Boosting – in predicting the risk associated with COVID-19 patients based on their symptoms, status, and medical history. This research is targeted at the process of patient state determination. The research subjects are machine learning methods for patient state determination. To achieve the aim of the research, the following tasks have been formulated: methods and models of the COVID-19 patients state determination should be analyzed; classification model of the patient state determination based on Bayes criterion should be developed; classification model of the patient state determination based on logistic regression should be developed; classification model of the patient state determination based on gradient boosting should be developed; the information system should be developed; the experimental study based on machine learning methods should be provided; and the results of the experimental study should be analyzed. Methods: using a dataset provided by the Mexican government, which encompasses over a million unique patients with 21 distinct features, we developed an information system in C# programming language. This system allows users to select their preferred method for risk calculation, offering a real-time decision-making tool for healthcare professionals. Results: All models demonstrated commendable accuracy levels. However, subtle differences in their performance metrics, such as sensitivity, precision, and the F1-score, were observed. The Gradient Boosting method slightly outperformed the other models in terms of overall accuracy. Conclusions: While each model showcased its merits, the choice of method should be based on the specific needs and constraints of the healthcare system. The Gradient Boosting method emerged as marginally superior in this study. This research underscores the potential of machine learning in enhancing pandemic response strategies, offering both scientific insights and practical tools for healthcare professionals. Пандемія COVID-19 поставила перед світовими системами охорони здоров'я безпрецедентні виклики, акцентуючи необхідність прогнозних інструментів для розподілу ресурсів та догляду за пацієнтами. Це дослідження занурюється в потенціал моделей машинного навчання для прогнозування оцінки ризику пацієнтів з COVID-19, використовуючи всеосяжний набір даних. Метою цього дослідження було оцінити та порівняти ефективність трьох методологій машинного навчання – Байєсівського критерію, логістичної регресії та градієнтного бустінгу – у прогнозуванні ризику, пов'язаного з пацієнтами з COVID-19, на основі їх симптомів, статусу та медичної історії. Об’єктом дослідження є процес визначення стану пацієнта. Предметом дослідження є методи машинного навчання для визначення стану пацієнта. Для досягнення мети дослідження були сформульовані наступні завдання: слід проаналізувати методи та моделі визначення стану пацієнтів з COVID-19; слід розробити класифікаційну модель визначення стану пацієнта на основі критерію Байєса; слід розробити класифікаційну модель визначення стану пацієнта на основі логістичної регресії; слід ти інформаційну систему; слід провести експериментальне дослідження на основі методів машинного навчання; слід проаналізувати результати експериментального дослідження. Методи: Використовуючи набір даних, наданий урядом Мексики, що охоплює понад мільйон унікальних пацієнтів з 21 відмінною ознакою, ми розробили інформаційну систему на мові програмування C#. Ця система дозволяє користувачам вибирати бажаний метод для розрахунку ризику, пропонуючи інструмент прийняття рішень у реальному часі для медичних фахівців. Результати: Усі моделі продемонстрували високі рівні точності. Однак були зауважені невеликі відмінності в їх показниках продуктивності, таких як чутливість, точність та показник F1. Метод градієнтного бустінгу трохи перевершив інші моделі за загальною точністю. Висновки: Хоча кожна модель продемонструвала свої переваги, вибір методу повинен базуватися на конкретних потребах та обмеженнях системи охорони здоров'я. Метод градієнтного бустінгу виявився дещо кращим у цьому дослідженні. Дослідження підкреслює потенціал машинного навчання у покращенні стратегій реагування на пандемію, пропонуючи як наукові інсайти, так і практичні інструменти для медичних фахівців.